:
Fine-Controllable and Expressive Freestyle Portrait
Animation
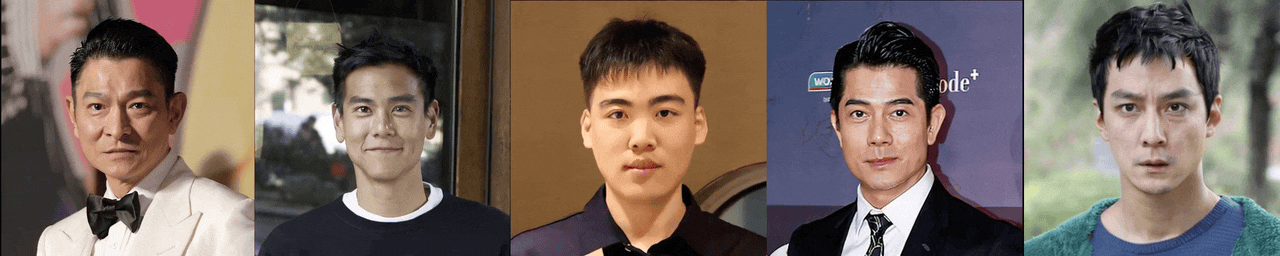
We present Follow-Your-Emoji, a diffusion-based framework for portrait animation, which animates a reference portrait with target landmark sequences. The main challenge of portrait animation is to preserve the identity of the reference portrait and transfer the target expression to this portrait while maintaining temporal consistency and fidelity.
To address these challenges, Follow-Your-Emoji equipped the powerful Stable Diffusion model with two well-designed technologies. Specifically, we first adopt a new explicit motion signal, namely expression-aware landmark, to guide the animation process. We discover this landmark can not only ensure the accurate motion alignment between the reference portrait and target motion during inference but also increase the ability to portray exaggerated expressions (i.e., large pupil movements) and avoid identity leakage. Then, we propose a facial fine-grained loss to improve the model's ability of subtle expression perception and reference portrait appearance reconstruction by using both expression and facial masks. Accordingly, our method demonstrates significant performance in controlling the expression of freestyle portraits, including real humans, cartoons, sculptures, and even animals. By leveraging a simple and effective progressive generation strategy, we extend our model to stable long-term animation, thus increasing its potential application value. To address the lack of a benchmark for this field, we introduce \textbf{EmojiBench}, a comprehensive benchmark comprising diverse portrait images, driving videos, and landmarks. We show extensive evaluations on EmojiBench to verify the superiority of Follow-Your-Emoji.
@article{ma2024followyouremoji,
title={Follow-Your-Emoji: Fine-Controllable and Expressive Freestyle Portrait Animation},
author={Ma, Yue and Liu, Hongyu and Wang, Hongfa and Pan, Heng and He, Yingqing and Yuan, Junkun and Zeng, Ailing and Cai, Chengfei and Shum, Heung-Yeung and Liu, Wei and others},
journal={arXiv preprint arXiv:2406.01900},
year={2024}
}